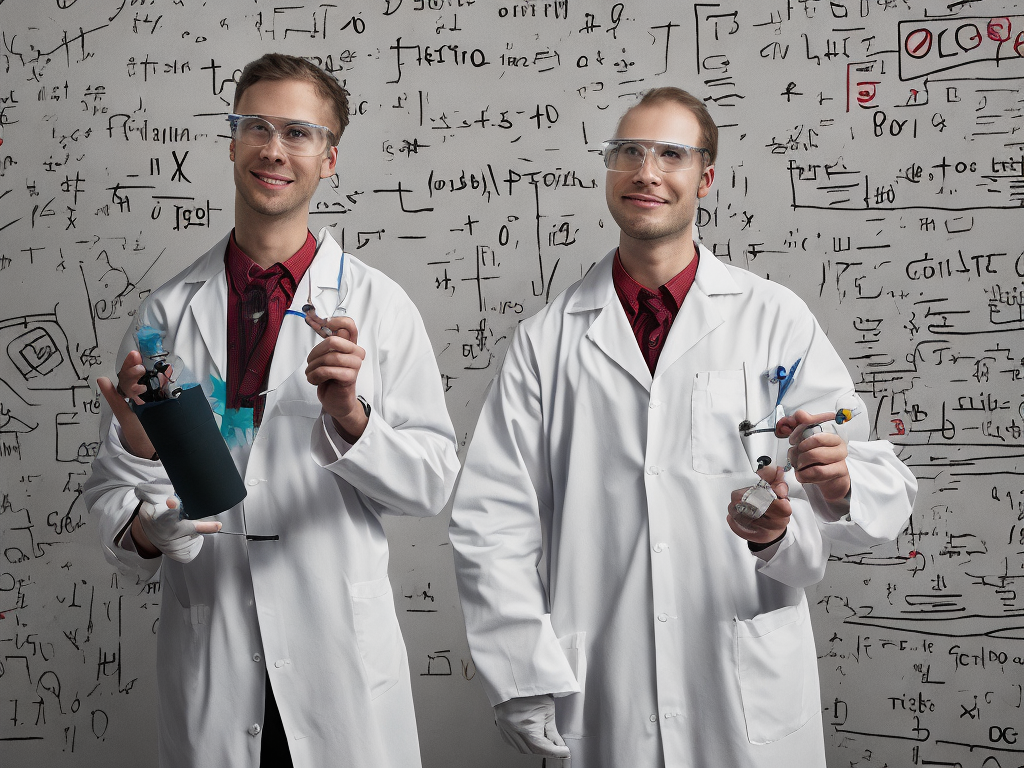
When analyzing data in statistical tests, researchers aim to draw accurate and reliable conclusions about a population based on the sample data they have. However, in statistical hypothesis testing, there is always a risk of making errors, which can lead to incorrect conclusions about the population. Statistical errors are classified into two broad categories, namely Type 1 error and Type 2 error. This article explains the differences between these two types of errors and their implications in research.
Type 1 error is also referred to as a false positive. A Type 1 error occurs if a researcher decides to reject the null hypothesis when it is true. In statistical testing, the null hypothesis is the assumption that there is no significant difference or relationship between the variables being tested. For example, if a researcher is investigating a new drug's effectiveness and the null hypothesis is that the drug has no effect, a Type 1 error occurs when the researcher concludes that the drug has an effect when, in reality, it does not. This means that the researcher concludes that there is a significant relationship between the two variables when there is none.
Type 1 error is also commonly known as a false positive. It is the error that comes up when the researchers reject the null hypothesis even though it is true. For instance, a hypothetical pharmaceutical company tests a new pain medication, and a random control group of 100 patients use it. When researchers analyze the results, they find that 10 patients report significantly reduced pain levels. Since the hypothesis stated that the medication worked, researchers conclude that the new pain medication works despite it actually having no effect on reducing pain levels.
Type 1 errors are more likely to occur if the significance level, also known as alpha, is set too low. The significance level is the probability of rejecting the null hypothesis when it is true. Common practice is to set the significance level at 0.05 (5%). This means that there is only a 5% chance of rejecting the null hypothesis when it is true. However, if the significance level is set too low, it increases the risk of Type 2 errors, where the null hypothesis is retained when it is false.
Type 2 error is also referred to as a false negative. A Type 2 error occurs if the researcher fails to reject the null hypothesis when it is false. In other words, this error occurs when the researcher fails to find a statistically significant difference when one actually exists. For example, if a researcher is investigating the effectiveness of a new cancer treatment and the null hypothesis states that the new treatment is no better than the standard treatment, the researcher would make a Type 2 error if they fail to detect that the new treatment is significantly better than the standard treatment.
Type 2 error is committed if the null hypothesis is accepted even though it is false. For instance, a COVID-19 researcher randomly selects 100 individuals to test for the virus. Assume that 10 have the virus. If the researcher concludes that none in the sample has COVID-19, the Type 2 error is committed, even though 10% of the sample had the virus.
Type 2 errors are more likely to occur if the sample size is too small or the effect size is too small. The effect size is the measure of the strength of the relationship between two variables under examination. A larger sample size or a larger effect size increases the ability of the statistical test to detect differences and reduce the risk of Type 2 errors.
While both Type 1 and Type 2 errors can lead to incorrect conclusions, they have different implications. Type 1 errors are generally considered more serious in research because they can lead to false discoveries, which can be costly, harmful, or dangerous. Suppose a pharmaceutical company rushes a drug to market based on a false discovery that the drug is effective. In that case, it can lead to severe side effects, health complications, or even lawsuits.
In contrast, Type 2 errors are often considered less serious in research because they are usually less harmful. Instead, they are likely to reduce statistical power, making it less likely to find significant differences or relationships between variables. However, Type 2 errors can also result in missed opportunities for discovering significant findings that may further scientific understanding or advance knowledge in a particular field.
The probability of a Type 1 or Type 2 error can be controlled by statistical power and sample size. Statistical power is the probability of rejecting the null hypothesis when it is false. It measures the ability of a test to detect significant differences or relationships between variables. A higher statistical power reduces the likelihood of committing Type 2 errors but increases the likelihood of committing Type 1 errors. Sample size also plays a crucial role in reducing the probability of errors since the larger the sample size, the greater the statistical power and the less likely to make errors.
In conclusion, Type 1 and Type 2 errors are common problems in statistical hypothesis testing, and they can lead to incorrect conclusions if not controlled. Type 1 errors occur when the researcher rejects a true null hypothesis, while Type 2 errors occur when the researcher fails to reject a false null hypothesis. While both errors can lead to incorrect conclusions, Type 1 errors are generally considered more serious in research. The probability of committing these errors can be reduced by controlling the significance level, statistical power, and sample size. It is important to understand the differences between these two types of errors to design robust research studies and avoid making incorrect conclusions based on sample data.