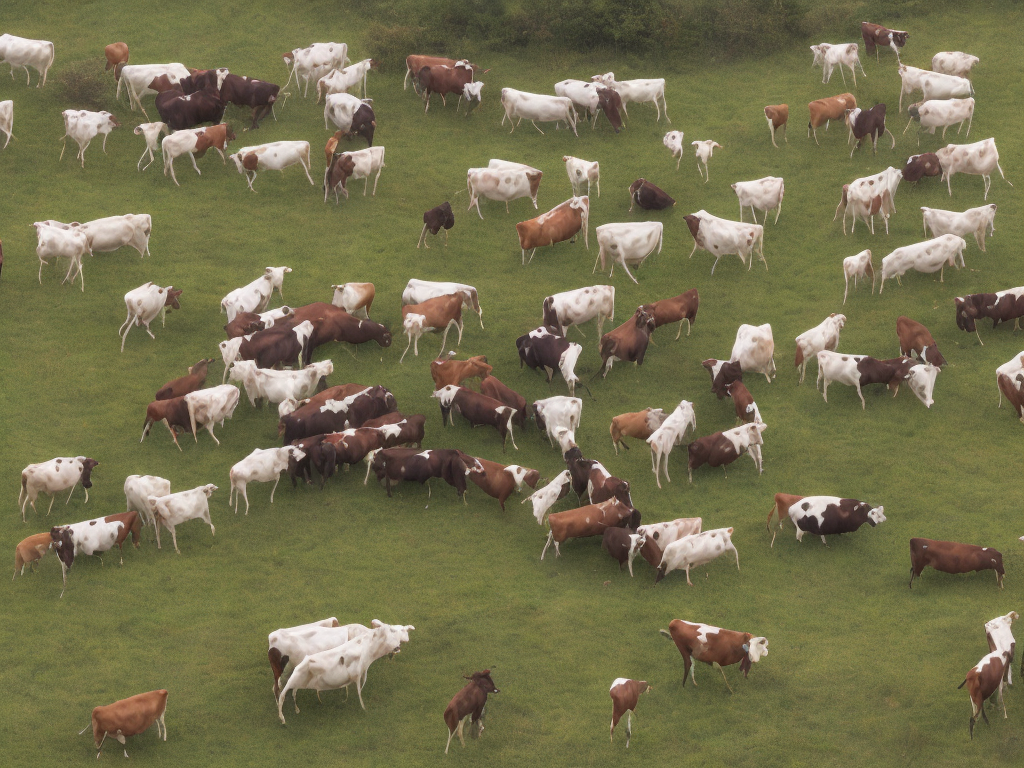
Statistics is the study of data and making meaningful conclusions and decisions based on the data. In the world of statistics, two terms that are often used are population and sample. In this article, we will explore and explain the difference between a population and a sample in statistics.
Population
In statistics, a population is defined as the entire group of individuals, objects, or events that are of interest in a study. It is the large group of elements from which a researcher collects data to make inferences and draw conclusions. For example, the population could be all people living in a particular country, all the cars in a city, or all the students in a school.
The population is often too large to collect data from every single individual, so researchers select a sample from the population to represent the population as a whole. The size of the population is an essential factor in determining the sample size.
Sample
A sample is a subset of the population selected for analysis. In contrast to the population, the sample is a smaller group of individuals, objects, or events from which a researcher collects data. Sampling is essential in statistics because it allows for the collection of data from a smaller group, which is manageable and more affordable.
The characteristics of the sample should be similar to those of the population to ensure the validity of the inferences drawn from the data collected. The size of the sample is also crucial in determining the accuracy of statistical estimates.
The difference between a population and a sample
In summary, the primary difference between a population and a sample is their size. The population is the entire group of individuals, objects, or events of interest, while the sample is a smaller group within the population, selected for analysis.
Another significant difference between the two is the type of analysis. The population is analyzed using descriptive statistics, while the sample is analyzed using inferential statistics.
Descriptive statistics are used to summarize and describe the characteristics of a population. Some of the measures that are used in this analysis include mean, median, mode, range, variance, and standard deviation. For example, the mean age of all students in a school is a descriptive statistic.
Inferential statistics is used to make inferences about the population based on the data collected from the sample. This type of analysis is used to test hypotheses and make predictions about the population. For example, the percentage of students who prefer online learning over traditional learning can be tested using inferential statistics.
Why the difference is important
The distinction between a population and a sample is essential in statistical analysis because it determines the accuracy of the conclusions and decisions made based on the data collected. Inaccurate sampling can lead to incorrect conclusions, which can significantly affect decisions made based on the data. For instance, a research study that investigates customer satisfaction regarding a product is more accurate if the sample is a true representation of the population.
Additionally, the size of the population and sample affects the accuracy of the statistical analysis. For instance, if a researcher wants to collect data from all students in the entire country, it will be impossible due to time and financial constraints. In such a scenario, the researcher may select a representative sample that will represent the population of interest. However, it's important to ensure that the sample is large enough and represents the characteristics of the population accurately.
Conclusion
In summary, understanding the difference between a population and a sample is a fundamental concept in statistical analysis. A population refers to the entire group of individuals, objects, or events of interest, while a sample is a smaller group of the population selected for analysis. The difference between the two is important because it determines the accuracy of the statistical analysis and the conclusions made based on the data collected.
The size of the population and sample also affects the accuracy of the analysis. Therefore, it is essential to ensure that the sample is large enough and represents the characteristics of the population accurately. By using the correct statistical tools and analysis, researchers can make confident inferences and conclusions about the population of interest.